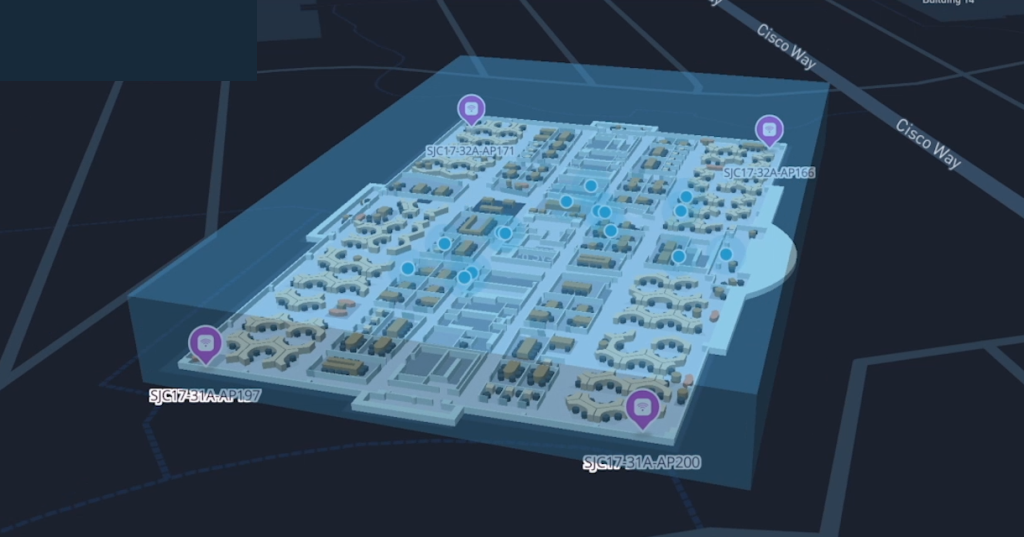
AP Auto Locate introduces a groundbreaking feature that empowers customers to precisely locate APs at the campus, building, and floor level, revolutionizing the management of wireless AP networks on a large scale. As shown in Figure 1, auto-locate utilizes a sophisticated building clustering algorithm to categorize APs into specific buildings within a campus environment. Once the APs are identified within their respective buildings, the system seamlessly transitions to the floor clustering algorithm, meticulously determining the exact floor where the desired APs are located.
Finally, AP Auto Locate employs a combination of GNSS raw data and ranging FTM (Fine Timing Measurement) data to position the APs with unparalleled precision onto the floor map. This integration of cutting-edge positioning technologies not only ensures the accuracy of AP placement but also facilitates seamless integration with existing floor maps, providing users with a comprehensive visual representation of their network infrastructure. In the following sections, we dive deeper into the intricate details of the AP positioning process explaining algorithms and methodologies employed to achieve unparalleled accuracy and reliability.

As shown in Figure 2, AP auto-locate uses two main sources of data to position the APs: 1) GNSS raw data and 2) the inter-AP distances.
GNSS raw data- Cisco AP auto-locate technology uses GNSS raw data. This data consists of 1) the time of the measurement, 2) satellite pseudoranges measured between the satellite and GNSS receiver, 3) their standard deviations, 4) carrier phase, and 5) Doppler shifts.
Inter-AP distance measurement (ranging)- uses 802.11mc/az also known as Fine Time Measurement (FTM) which involves leveraging precise time measurements to determine the distance between two points or objects. This technique relies on accurately measuring the time it takes for a signal or wave to travel from a transmitter to a receiver and back again.
Cisco APs are equipped with GNSS receivers. GNSS raw data is collected from APs located on the perimeter of buildings ensuring optimal azimuthal coverage or skyview. Using the inter-AP distances (measured by FTM), these APs are used as anchors in the positioning process, facilitating the precise positioning of other APs that lack GNSS receivers or suffer from inadequate satellite coverage.
In scenarios where GNSS reception is unavailable or insufficient, AP Auto Locate seamlessly transitions to utilizing ranging data to calculate the relative positions of APs, ensuring accuracy and reliability in AP localization across diverse environmental conditions. Further, the customers are equipped with a drag-and-drop functionality, allowing them to fine-tune the AP constellation directly on the map interface.
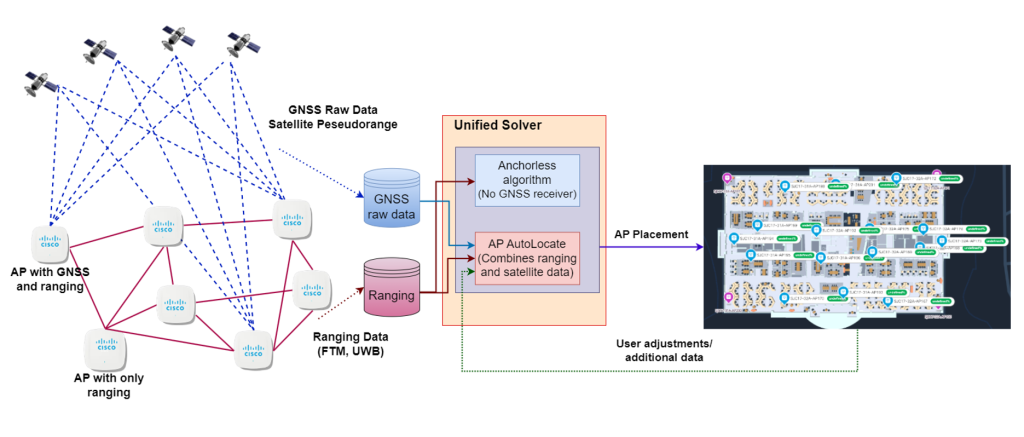
Conventional methods rely solely on the calculated positions provided by GNSS receivers, rather than utilizing GNSS raw data directly. In this approach, the positions of APs calculated with GNSS receivers act as fixed anchors, and ranging data is integrated to determine the positions of other APs within the network. However, this methodology is susceptible to error propagation, wherein the inaccuracies associated with each GNSS receiver’s calculated position are compounded, leading to significant inaccuracies in the overall AP positioning. As shown in Figure 3 the reliance on calculated positions without GNSS raw data leads to error accumulation and in suboptimal localization.
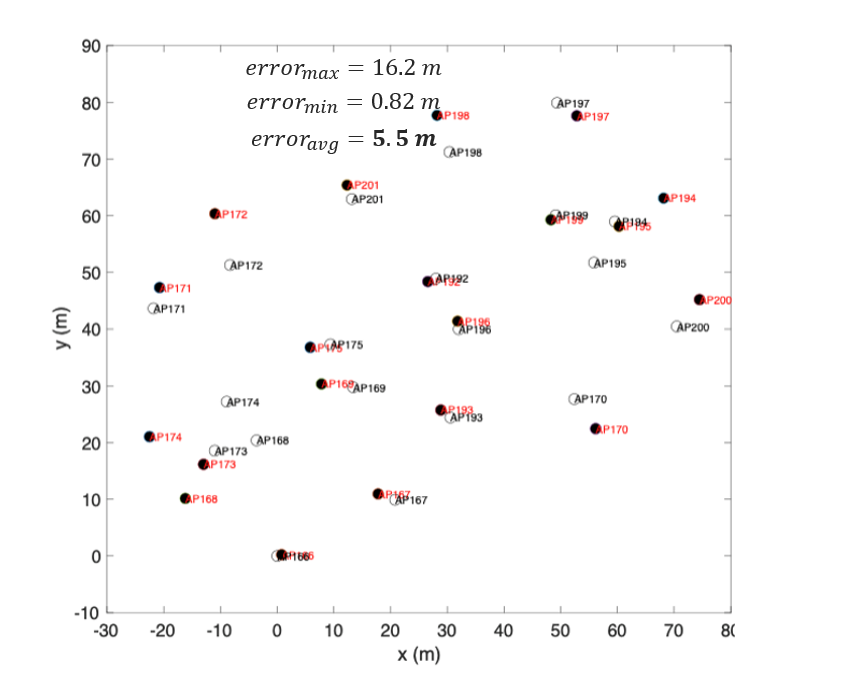
In contrast, our innovative approach adopts a more advanced methodology that utilizes GNSS raw data alongside the precise location information of satellites as fixed anchors. By integrating this comprehensive dataset with ranging data, our method accurately determines the positions of APs with much higher accuracy. As shown in Figure 4, the integration of GNSS raw data and satellite location information enhances the precision of AP positioning significantly. By leveraging this robust combination of data sources, our method mitigates error propagation and significantly improves the overall accuracy and reliability of AP localization.
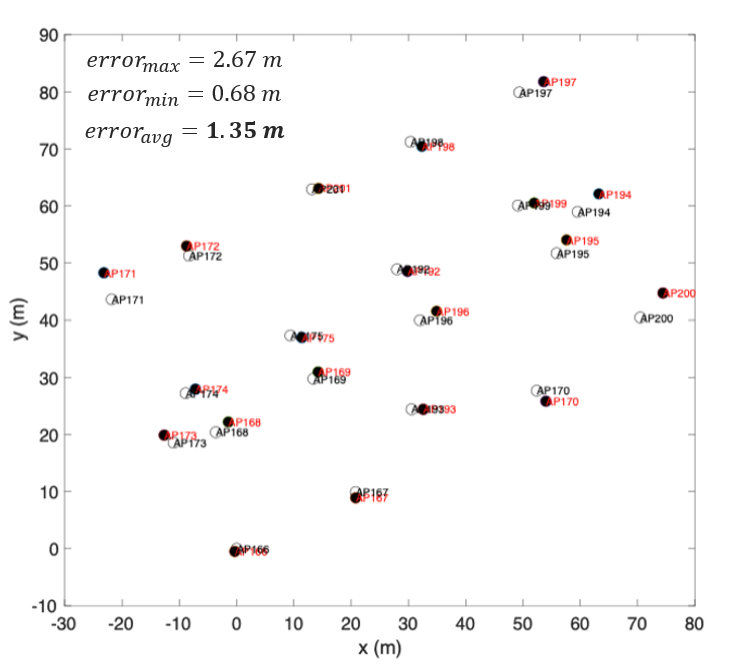
This is an example in our testbed in a Cisco building in San Jose.
The precision of the location is contingent upon several factors, including the building construction materials, the spacing between access points (APs), and the geographical location of the building itself.
The canyon effect can reduce GNSS accuracy. The “canyon effect”, also known as the urban canyon, is where tall, packed buildings surround a street, in turn, creating an artificial canyon effect. If you’re down in a valley surrounded by mountains, you might not be able to see enough sky above you to contain four satellites.
Furthermore, the accuracy of Fine Timing Measurement (FTM) is influenced by the relative permittivity of construction materials.
Advantages of AP Auto Locate
AP auto-locate offers a trifecta of compelling advantages that revolutionize the management of wireless APs and indoor location services. Foremost, it facilitates the tedious task of setting up new sites by seamlessly and accurately positioning APs without the need for labour-intensive and error-prone manual intervention. This transformative feature is particularly invaluable in new site addition or site expansion scenarios (Greenfield), where hundreds of APs must be precisely placed concurrently. By automating this process, AP auto-locate drastically reduces deployment time and costs while ensuring accurate AP placement for better network visibility and precise indoor location services.
Moreover, AP auto-locate empowers IT specialists with enhanced remote management capabilities, allowing them to effortlessly monitor APs via detailed maps. This remote monitoring functionality enables real-time detection of any AP movements, hardware additions, or removals, facilitating proactive troubleshooting and maintenance without the necessity of physical site visits. Knowing the precise position of the Aps, IT teams can swiftly locate impacted APs, address issues, optimize network performance, and ensure uninterrupted connectivity.
Finally, AP auto-locate delivers accuracy in AP positioning, a critical requirement for robust indoor location services. By precisely determining AP positions, it enables reliable people and asset tracking within indoor environments, laying the foundation for several applications from navigation assistance to inventory management. The precise positioning of APs serves as the anchor for locating other indoor assets using trilateration, ensuring high accuracy in indoor navigation and monitoring capabilities.
About the Author:
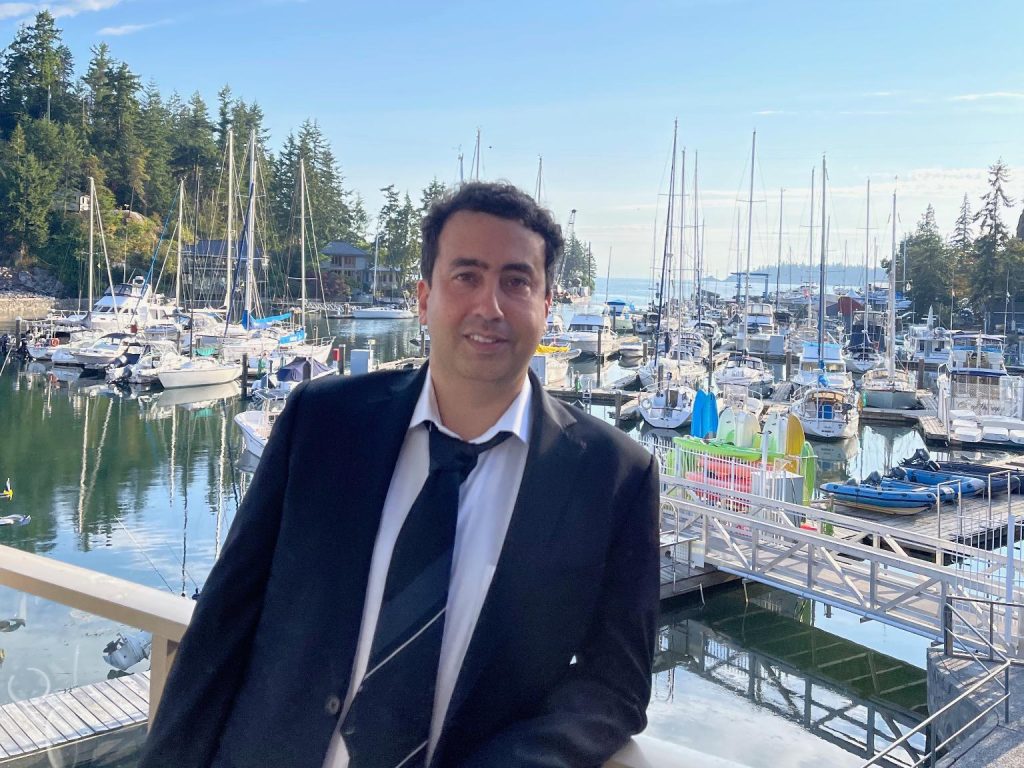
Peiman has a PhD degree from university of Utah in wireless communications. After his PhD, he worked as Sr. Staff Scientist at Broadcom for 5 years where he made contributions to multiple 11n and 11ac chipset designs as well as IEEE 802.11 and WFA standard development. After Broadcom, he joined Netgear where he managed the system architecture team for more than 6 years.
At Netgear, he designed several Wi-Fi access and Camera products including Netgear wireless routers (Nighthawk), wireless mesh systems (Orbi), and wireless security cameras (Arlo). After Netgear, Peiman joined ON Semiconductor as Director of Product design and was in charge of a client combo chip designed for automotive applications . Peiman also worked on mmWave fixed wireless access for service providers.
At Cisco, Peiman has been a principal software architect for Cisco’s CW APs including CW9166, CW9164, and CW9162. The CW APs enable Cisco customers to choose between on premises and cloud management. Moreover, Peiman founded OpenAFC along with Meta and Broadcom. OpenAFC is being used for majority of AFC submissions including WiFi Alliance, Wireless Broadcom Alliance, and getting certified by US Government (FCC) and Canadian Government (ISED). Peiman is currently leading the location effort in wireless access point team on AP Auto locate, Client location(FTM, UWB, HADM). The AP self locate is key feature to simplify the deployment process for customers which need a map and has received great feedback from Cisco customers.
Peiman has over 120 issued patents in wireless communications, signal processing, and image processing and has been one of the top innovators in Wi-Fi industry.